The amount of data being captured in today’s digitized world is simply staggering. Google conducts about 4 million searches, 180 million emails are being sent, and 1 million people log into Facebook every single minute. On top of that, the data volume being produced is increasing exponentially. It has been estimated that 90% of the worlds data has been produced in the last 2 years.
These massive data volumes are at the foundation of today’s AI hype. Success stories from the tech giants have shown that there can be tremendous value hidden in these datasets. The good news is that you don’t always need billions of data points to get value out of your data. Smaller companies can unlock this value as well, because the most important piece of the puzzle is not the billion data rows or the advanced AI algorithm. The most important part is defining a good use case to improve your business processes and to serve your customers better. These use cases can be different for every business, but to get you started, we have created a list of the top 5 data use cases in CRM.
Interactive reporting
With the recent hype around AI, the value of good reporting is often forgotten. An interactive dashboard is most likely the quickest way to get value out of your data. We’re not talking about a static Excel file emailed to the entire company once a month. To get real value out of reporting you need to develop self service dashboards, where the end users can drill down into the data by themselves to find the detailed answers they are looking for. These dashboards can cover a wide range of use cases, such as analysing your sales pipeline, your campaign effectiveness, etc. If you are using Salesforce as your CRM system, you can utilise the Einstein Analytics platform to achieve this interactive reporting natively within the system you use on a daily basis.
Improve customer retention
Churn modelling is probably the most popular data science use case in CRM. The idea is simple: we want to know which customers are loyal, and which customers are at risk of leaving our business. To achieve this we typically use a technique called ‘predictive modelling’, in which we will train a model on historical data (which customers have left our business in the past?) and apply it on our existing customer base (who is most likely to leave next?). Knowing which customers are most likely to churn can massively improve the effectiveness of your retention campaigns. This use case is most obvious for subscription based businesses, but other businesses such as retailers can also use it to predict for example which customers are most likely to stop visiting their stores.
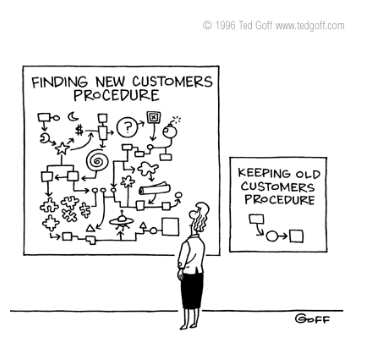
Identify sales opportunities
We can use the same predictive modelling techniques not only to identify who is most likely to churn, but also to identify who is most likely to buy new or additional products. Your sales reps might be working on hundreds or even thousands of leads and opportunities. Knowing which leads are most likely to convert enables your sales reps to focus on the deals that really matter.
Optimize customer service
Many customer service departments nowadays are receiving more and more incoming messages. It’s not always possible to increase the support staff to deal with these larger volumes. On top of that, most messages are often very repetitive and employee turnover is typically very high when agents spend most of their time on these repetitive tickets. In order to optimize your customer service, you can use Natural Language Processing to assist agents in solving repetitive cases faster. An AI model can automatically identify a customer’s intent, classify the case and prompt a relevant response to the agent. Handling time for repetitive tickets will drastically decrease, which leads to a better customer experience as well as a better employee experience.
Forecast product demand and optimise stock levels
Optimizing the logistic process for retailers can be very challenging. On the one hand they want to have enough inventory to avoid stock outs, but on the other hand keeping too much inventory is very expensive. Predicting demand and ordering the right amount of inventory based on gut feeling is very hard when you have many products and many different locations. These tasks are very well suited for an AI model. It can analyse your historical sales data and generate accurate forecasts of future demand for thousands of individual products. Based on that product demand you can optimize your inventory levels to reduce holding and transportation costs.
Can you think of any other use cases that would benefit your business? Not quite sure about where to start? Get in touch and we’ll be happy to discuss!